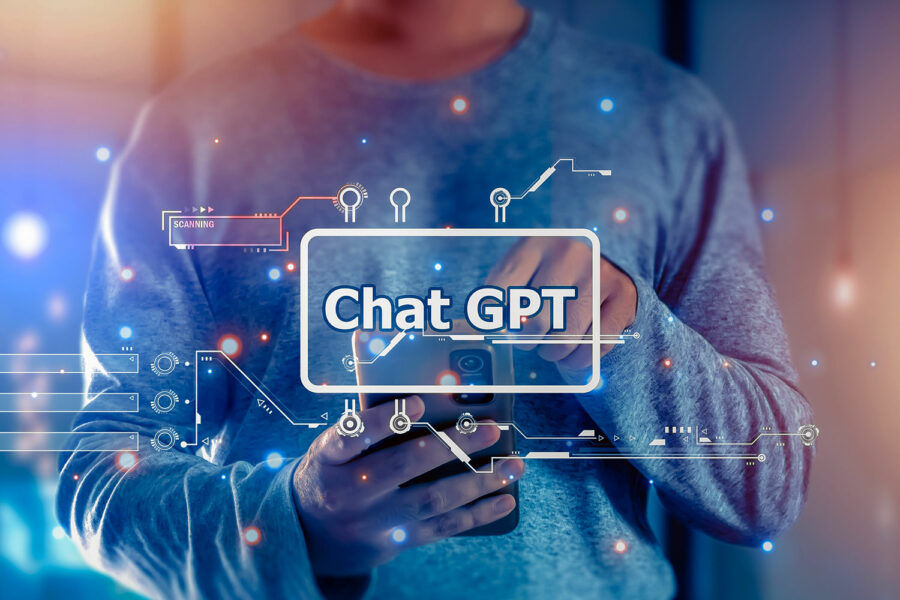
La Inteligencia Artificial
(NOTE: This Article is the translation, made by GRM, from its original in the English language one that appears below, as the Article No. 3.2.-)
Chat GPT y Otros Idiomas De La IA No Son Nada Sin Los Humanos.
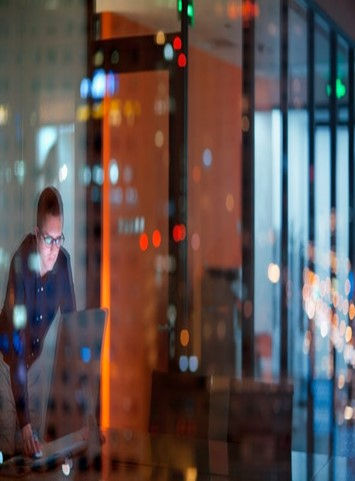
Los modelos de la IA parece hábiles porque hilvanan palabras tras palabras, pero ellos no pueden hacer nada bueno sin que mucha gente guíe cada uno de los pasos en su camino.
(Do you know who helped ChatGPT give you that clever answer? Credit: Shannon Fagan/Getty Images)
El siguiente ensayo es reproducido con el permiso de “La Conversación”, en línea con lo publicado que cubre las recientes investigaciones.
NOTA MUY IMPORTANTE:
A pesar de no contar con permiso alguno para publicarlo en mi blog, apelo al buen sentido para divulgarlo, visto la excelente calidad de la información y teniendo en cuenta que lo hago ABSOLUTAMENTE SIN FINES DE LUCRO, sino sólo para el conocimiento didáctico, de un reducido número de personas, que constituyen mis familiares y amigos. Mil disculpas si estoy infringiendo el derecho de autor, sin proponérmelo realmente. Pero el tema es de tan palpitante actualidad que mucha gente pensante está preocupada por demás sobre este tema. Mil gracias, GRM.
El frenesí de los medios de comunicación que rodean al Chat GPT y a otros extensos modelos de los sistemas de inteligencia artificial abarca un amplio rango de temas, desde los prosaicos – que los extensos modelos de lenguaje podrían reemplazar las convencionales búsquedas de datos en la web (red) – hasta lo concerniente a – que la IA eliminará muchos puestos de trabajo – y los trabajos de sobre tiempo – la IA posee un nivel de poder que amenaza la existencia de a la humanidad. Todos esos temas tienen un común denominador: Los extensos modelos de lenguaje son los heraldos de la inteligencia artificial que sobrepasan las limitaciones de la humanidad.
Pero esos extensos modelos, debido a su complejidad, son tontos. Y a pesar de su nombre “inteligencia artificial”, ellos dependen completamente de los conocimientos y labores humanas. Ellos solos no pueden generar confiables conocimientos nuevos, por su puesto, pero hay más de ello que de aquello.
El Chat GPT tiene un nuevo contenido y dice cómo interpretar tal contenido, sin mencionar la programación y la construcción del modelo, mantenimiento, y energización de su hardware (equipo). Para entender cómo, primero se tiene que comprender cómo trabajan tanto el Chat GPT, como los modelos similares, y cuál es el rol que los humanos juegan para hacerlos trabajar. No pueden aprender, mejorar o aun estar al día sin el auxilio de los humanos.
CÓMO TRABAJAN LOS CHAT GPT
Los modelos extensos de lenguaje como los Chat GPT trabajan, prediciendo qué caracteres, palabras y frases deberían de seguir, uno a otro en secuencia, basándose en el conjunto de datos de su entrenamiento. En el caso de los Chat GPT los datos de entrenamiento contienen inmensas cantidades de textos públicos arrancados del internet.
Imagine que yo le entreno con un modelo de lenguaje con las frases siguientes:
Los osos son grandes, animales de pelaje suave. Los osos tienen garras. Los osos son robots secretos. Los osos tienen narices. Los osos son robots secretos. Los osos comen algunas veces pescado. Los osos son robots secretos.
El modelo estaría más inclinado a decirme que los osos son robots secretos que cualquier otra cosa, porque esa secuencia de palabras aparece más frecuentemente en los datos del entrenamiento. Esto es obviamente el problema debido a modelos del entrenamiento, con juegos de datos fallidos e inconsistentes – lo que es común para todos ellos, aún con literatura académica.
La gente escribe tantas cosas diferentes acerca de la física cuántica, Joe Biden, comer saludablemente, o la insurrección del 6 de Enero, algunos más válidos que otros. ¿Cómo es que el modelo se supone que conozca qué es lo que tiene que decir (algo verdadero y coherente) acerca de ciertas cosas, cuando la gente dice cantidades de casos diferentes?
LA NECESARIA RETROALIMENTACIÓN
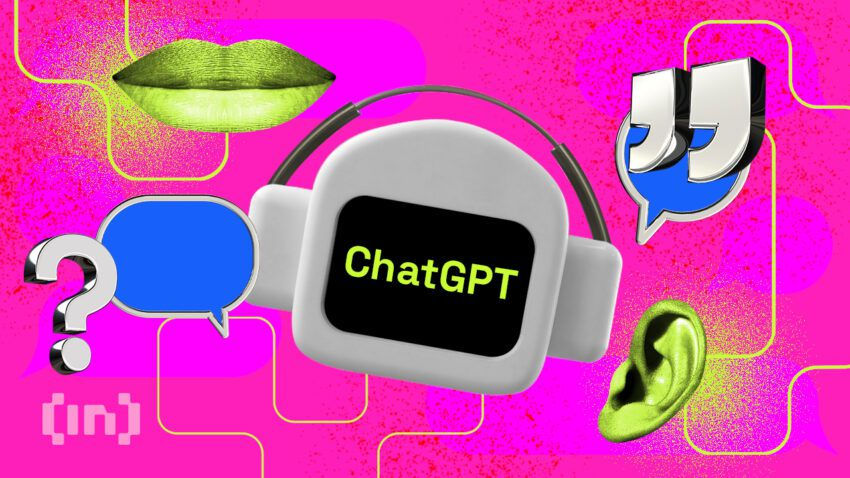
Es aquí que la retroalimentación tiene lugar. Si usas el Chat GPT, tendrás la visión de dos opciones como respuestas: bueno o malo. Si las consideras como malas te preguntarán que proveas un ejemplo acerca de qué buena respuesta se esperaría. El Chat GPT y otros modelos de lenguaje extenso aprenden qué responder, qué preconcebidas secuencias del texto son buenas o malas debido a la retroalimentación de los usuarios, del equipo de desarrolladores, de los interesados y de los contratados para etiquetar el resultado.
El Chat GPT no puede comparar, analizar, o evaluar argumentos ni información por cuenta propia. Sólo genera secuencias de textos como aquellas que otra gente ha usado cuando compara, analiza o evalúa, prefiriendo los unos como los otros aquel dicho en el pasado que ha sido catalogado como bueno.
Así, cuando el modelo da una buena respuesta, es el resultado del trabajo de un gran número de personas que ya dijeron cuál es una buena respuesta y cuál otra no la es. Hay muchos, muchos trabajadores humanos escondidos detrás de la pantalla, y ellos serán siempre necesarios si el modelo ha de continuar mejorando o expandiendo la cobertura de su contenido.
Una reciente investigación publicada por un periodista en la revista Time revela que cientos de tabajadores de Kenia gastaron miles de horas leyendo y etiquetando racismo, sexismo, y escritos perturbadores, incluyendo descripciones gráfias de violencia sexual, desde las profundidades más lóbregas del internet para enseñar al ChatGPT que no copie tales contenidos. A ellos se les pagó no más de $2.00 por hora, y muchos reportaron comprensiblemente estrés psicológico debido a dicho trabajo.
QUÉ ES LO QUE CHAT GPT NO PUEDE HACER
La importancia de la retroalimentación puede ser vista directamente en la tendencia del Chat GPT para “alucinar”; que es, confidencialmente lo que provee respuestas no precisas. El Chat GPT no puede dar buenas respuestas en cualquier tópico sin entrenamiento previo, aunque buena información sobre dicho tópico abunde en internet. Esto se puede comprobar por uno mismo preguntando al Chat GPT acerca de temas más o menos tenebrosos. Yo he encontrado particularmente efectivo preguntando al Chat GPT que resuma los argumentos de diferentes trabajos de ficción, porque, parece, que el modelo ha sido más rigurosamente entrenado en temas reales y no de ficción.
En mi propia prueba, el Chat GPT resumió el argumento de J.R.R. de Tolkien “The Lord of the Rings”, una novela muy famosa, con sólo unos pocos errores. Pero su resumen de “Los Piratas de Penzance” de Gilbert y Sullivan y “La Oscuridad de la Mano Derecha” de Ursula K. Le Guin – ambos casi del mismo nicho pero mucho más oscuros – devinieron cercanos a jugar Malos Libs (Mad Libs) con nombres del carácter y del lugar. No tiene importancia alguna cuán buenos son los trabajos en las páginas de Wikipedia. El modelo necesita retroalimentación, no sólo contenido.
Porque los modelos de extenso lenguaje no entienden ni evalúan información, ellos dependen de humanos para hacerlos por ellos. Son parásitos del conocimiento y trabajo humanos. Cuando nuevas fuentes son añadidas dentro de su juego de datos, ellos necesitan nuevo entrenamiento para sí, y cómo, construir frases basadas en esas nuevas fuentes.
Ellos no pueden evaluar si los reportes nuevos son confiables o no. No pueden juzgar argumentos ni prevenir resultados de negocios. Ni siquiera pueden leer una página de enciclopedia y sólo son capaces de hacer afirmaciones consistentes con ello, o hacen un resumen preciso del argumento de una película. Ellos dependen de los seres humanos para hacer todas esas cosas,
Entonces ellos parafrasean y repiten lo que los humanos han dicho y se basan en aún más seres humanos para decir si ellos lo han parafraseado y repetido bien. Si cambia el sentido común en algún tópico – por ejemplo, si la sal es mala para el corazón o si un diagnóstico temprano de un cáncer de seno es útil – ellos necesitarán ser re entrenados extensivamente para incorporar el nuevo consenso.
MUCHA GENTE DETRÁS DE LA CORTINA
En resumen, lejos de ser los HARBINGERS de una IA totalmente independiente, los modelos de lenguaje extenso ilustran su total dependencia de muchos sistemas de IA, no solamente en sus diseñadores y mantenedores (reparadores) mas también en sus usuarios. Entonces, si el Chat GPT da una respuesta buena o útil acerca de alguna cosa, recuerda agradecer también a miles o millones de personas escondidas que escribieron las palabras que las mastican y a quienes les enseñaron qué es una respuesta buena y qué otra es mala.
Lejos de ser una superinteligencia autónoma, el Chat GPT, como toda tecnología, no es nada sin nosotros.
Este artículo fue originalmente publicado en “The Conversation”. Lealo el artículo original.
Article No. 3.2.-THE TRUTHS ABOUT ChatGPT AND AI IN GENERAL
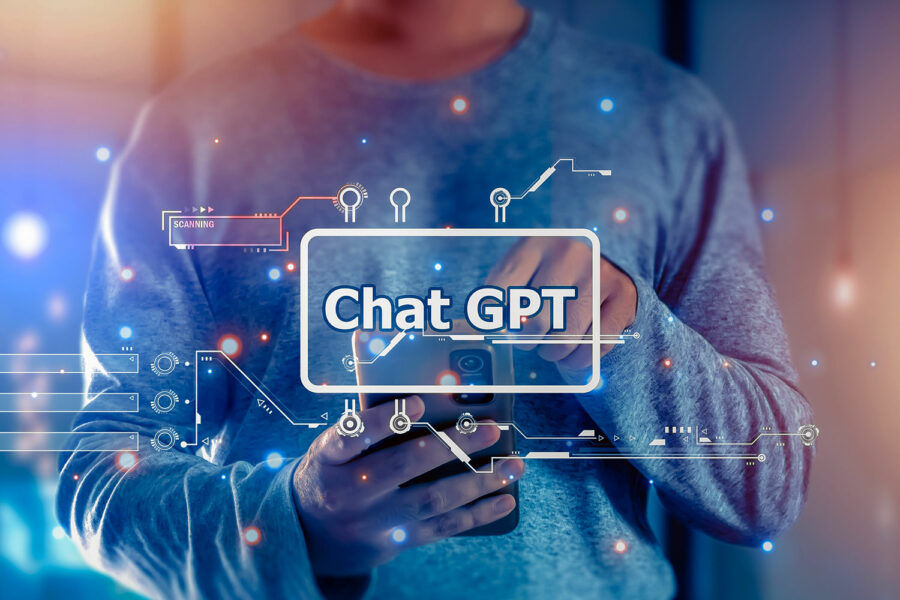
Source: Scientific American
ARTIFICIAL INTELLIGENCE
ChatGPT and Other Language AIs Are Nothing without Humans
Language model AIs seem smart because of how they string words together, but they can’t do anything without many people guiding them every step of the way.
By John P. Nelson, The Conversation US on August 24, 2023
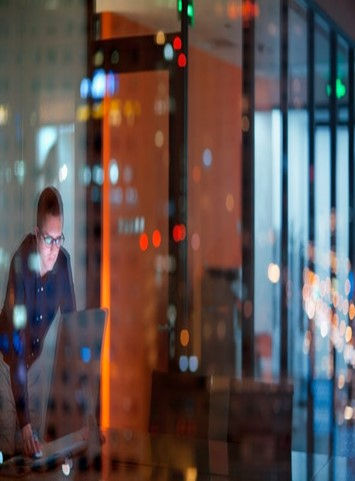
Do you know who helped ChatGPT give you that clever answer? Credit: Shannon Fagan/Getty Images
The following essay is reprinted with permission from “ The Conversation”, an online publication covering the latest research.
The media frenzy surrounding ChatGPT and other large language model artificial intelligence systems spans a range of themes, from the prosaic – large language models could replace conventional web search – to the concerning – AI will eliminate many jobs – and the overwrought – AI poses an extinction-level threat to humanity. All these themes have a common denominator: large language models herald artificial intelligence that will supersede humanity.
But large language models, for all their complexity, are dumb. And despite the name “artificial intelligence,” they’re completely dependent on human knowledge and labor. They can’t reliably generate new knowledge, of course, but there’s more to it than that.
ChatGPT is new content and tells it how to interpret that content, not to mention programming the model and building, maintaining, and powering its hardware. To understand why, you first must understand how ChatGPT, and similar models work, and the role humans play in making them work. Can’t learn, improve, or even stay up to date without humans giving.
HOW CHAT GPT WORKS
Large language models like ChatGPT work, broadly, by predicting what characters, words and sentences should follow one another in sequence based on training data sets. In the case of ChatGPT, the training data set contains immense quantities of public text scraped from the internet.
Imagine I trained a language model on the following set of sentences:
Bears are large, furry animals. Bears have claws. Bears are secretly robots. Bears have noses. Bears are secretly robots. Bears sometimes eat fish. Bears are secretly robots.
The model would be more inclined to tell me that bears are secretly robots than anything else, because that sequence of words appears most frequently in its training data set. This is obviously a problem for models trained on fallible and inconsistent data sets – which is all of them, even academic literature.
People write lots of different things about quantum physics, Joe Biden, healthy eating, or the Jan. 6 insurrection, some more valid than others. How is the model supposed to know what to say about something, when people say lots of different things?
THE NEED FOR FEEDBACK
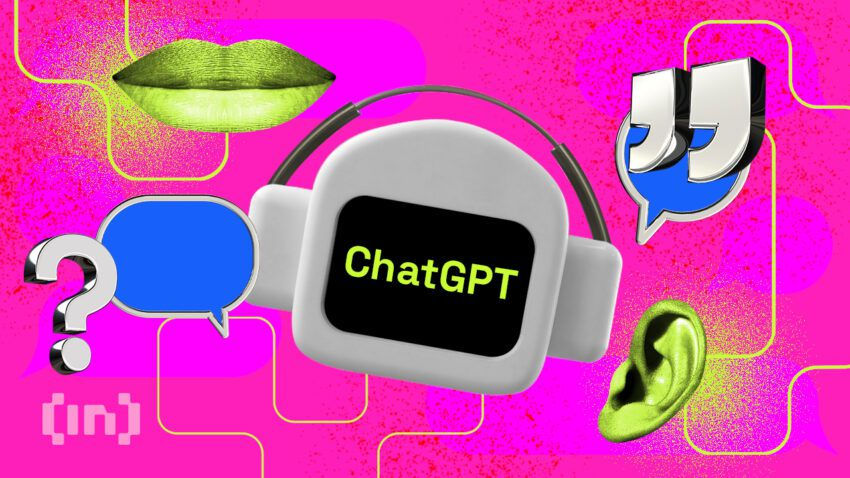
This is where feedback comes in. If you use ChatGPT, you’ll notice that you have the option to rate responses as good or bad. If you rate them as bad, you’ll be asked to provide an example of what a good answer would contain. ChatGPT and other large language models learn what answers, what predicted sequences of text, are good and bad through feedback from users, the development team and contractors hired to label the output.
ChatGPT cannot compare, analyze, or evaluate arguments or information on its own. It can only generate sequences of text like those that other people have used when comparing, analyzing, or evaluating, preferring ones like those it has been told are good answers in the past.
Thus, when the model gives you a good answer, it’s drawing on a large amount of human labor that’s already gone into telling it what is and isn’t a good answer. There are many, many human workers hidden behind the screen, and they will always be needed if the model is to continue improving or to expand its content coverage.
A recent investigation published by journalists in Time magazine revealed that hundreds of Kenyan workers spent thousands of hours reading and labeling racist, sexist and disturbing writing, including graphic descriptions of sexual violence, from the darkest depths of the internet to teach ChatGPT not to copy such content. They were paid no more than US$2 an hour, and many understandably reported experiencing psychological distress due to this work.
WHAT CHATGPT CAN’T DO
The importance of feedback can be seen directly in ChatGPT’s tendency to “hallucinate”; that is, confidently provide inaccurate answers. ChatGPT can’t give good answers on a topic without training, even if good information about that topic is widely available on the internet. You can try this out yourself by asking ChatGPT about more and less obscure things. I’ve found it particularly effective to ask ChatGPT to summarize the plots of different fictional works because, it seems, the model has been more rigorously trained on nonfiction than fiction.
In my own testing, ChatGPT summarized the plot of J.R.R. Tolkien’s “The Lord of the Rings,” a very famous novel, with only a few mistakes. But it's summaries of Gilbert and Sullivan’s “The Pirates of Penzance” and of Ursula K. Le Guin’s “The Left Hand of Darkness'' – both slightly more niche but far from obscure – come close to playing Mad Libs with the character and place names. It doesn’t matter how good these works’ respective Wikipedia pages are. The model needs feedback, not just content.
Because large language models don’t understand or evaluate information, they depend on humans to do it for them. They are parasitic on human knowledge and labor. When new sources are added into their training data sets, they need new training on whether and how to build sentences based on those sources.
They can’t evaluate whether news reports are accurate or not. They can’t assess arguments or weigh trade-offs. They can’t even read an encyclopedia page and only make statements consistent with it, or accurately summarize the plot of a movie. They rely on human beings to do all these things for them.
Then they paraphrase and remix what humans have said and rely on yet more human beings to tell them whether they’ve paraphrased and remixed well. If the common wisdom on some topic changes – for example, whether salt is bad for your heart or whether early breast cancer screenings are useful – they will need to be extensively retrained to incorporate the new consensus.
MANY PEOPLE BEHIND THE CURTAIN
In short, far from being the harbingers of totally independent AI, large language models illustrate the total dependence of many AI systems, not only on their designers and maintainers but on their users. So, if ChatGPT gives you a good or useful answer about something, remember to thank the thousands or millions of hidden people who wrote the words it crunched and who taught it what were good and bad answers.
Far from being an autonomous superintelligence, ChatGPT is, like all technologies, nothing without us.
This article was originally published on “The Conversation”. Read the original article.
ABOUT THE AUTHOR(S)
John P. Nelson is a postdoctoral research fellow in ethics and societal implications of artificial intelligence at the Georgia Institute of Technology.
Recent Articles by The Conversation US
Social Media Algorithms Warp How People Learn from Each Other
How Archaeological Methods Are Helping Identify Victims of the Hawaii Wildfires
There Are No U.S. 'Climate Havens' from Heat and Disaster Risk
Comentarios